Custom Dataset (Middle format, 포멧중립)
MMDetectio 논문 https://arxiv.org/pdf/1906.07155.pdf
https://github.com/open-mmlab/mmdetection
- Custom Dataset구조를 활용하여 새로운Dataset을 정의
- Config를 수정
- 새로운 detector를 학습한다.
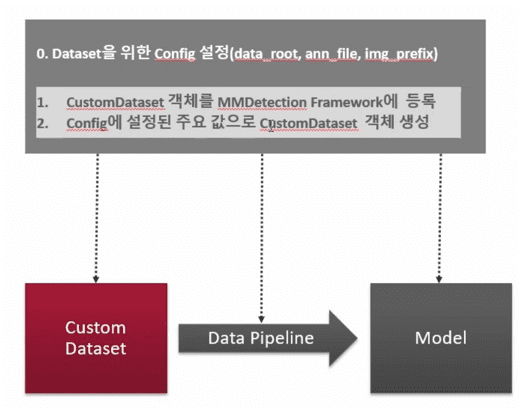
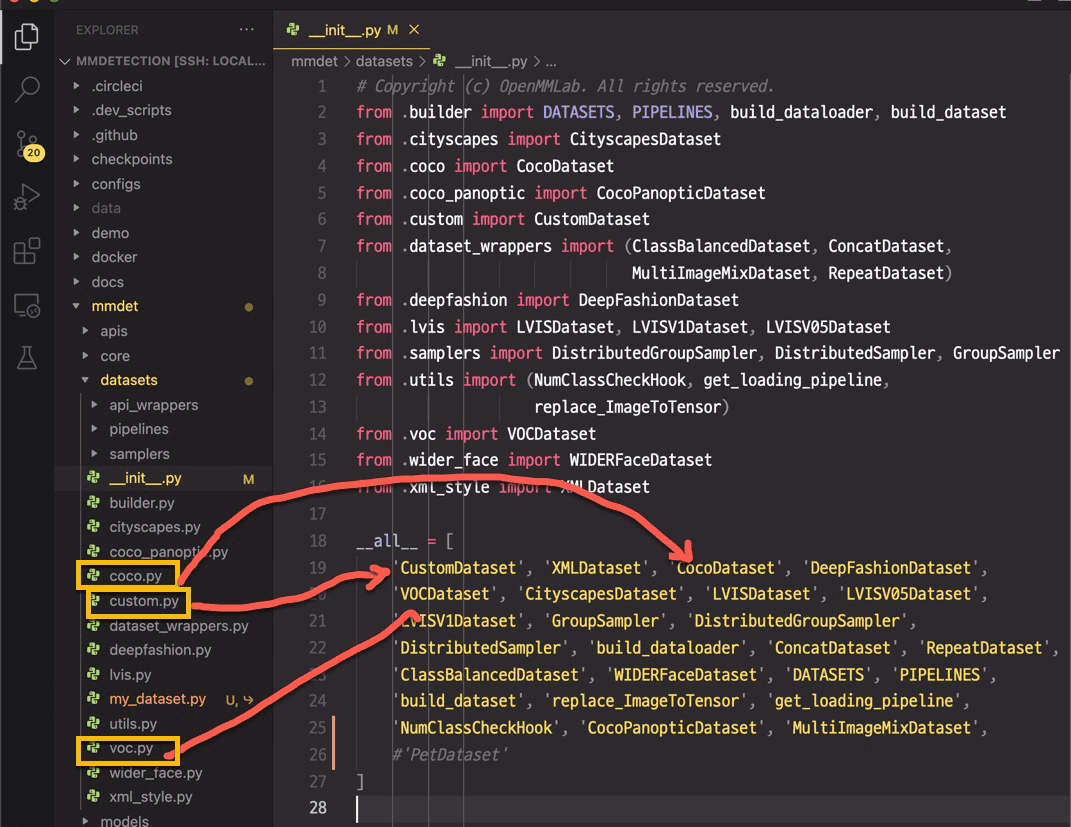
Custom Dataset으로 변경하여 메모리에 Load
Input Dataset구조를 Custom Dataset 구조로 변경
https://github.com/open-mmlab/mmdetection/blob/master/demo/MMDet_Tutorial.ipynb 의 발췌
- 모든 Image에 대해 Annotation정보를 단 1개의 list 객체로 (메모리에) 가짐
=> list내에 원소는 1개의 Image에 대해 dictionary형태로 구성
(즉, Image갯수만큼의 dictionary를 만듦) - ann내에 bbox는 2D array , label은 1D array
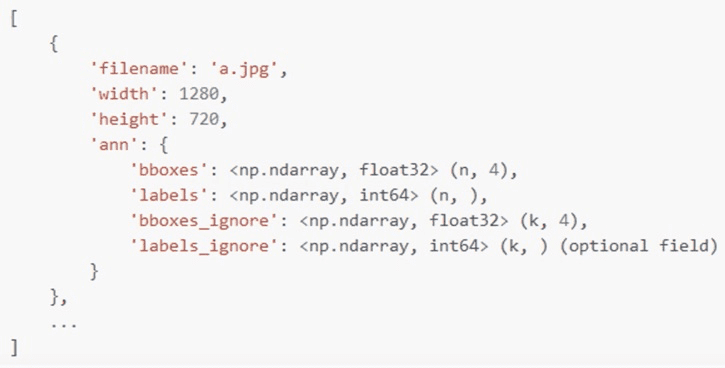
- filename: 이미지 파일명 (디렉토리없이)
- width: 이미지 너비
- height: 이미지 높이
- ann: bbox와 label(gT)에 대한 정보를 가지는 Dictionary
- bboxes: n개의 Object들 , 4개의 좌표값(좌상단, 우하단)을 갖는 numpy array. shape는 (n, 4)
- labels: n개의 Object들에 대한 numpy array. shape는 (n, )
- bboxes_ignore: 학습에 사용되지 않고 무시하는 k개의 bboxes. shape는 (k, 4)
- labels_ignore: 학습에 사용되지 않고 무시하는 k개의 labels. shape는 (k,)
Custom Dataset코드 ===> PipeLine ===> Model
- 아래 코드를 통해 CustomDataset 객체가 mmDetection 프레임웤에 등록되고, register_module()
- Config의 설정값(소스데이터 구조에 관한 data_root, img_prefix, ann_file)으로 객체가 생성되면, build_dataset()
- mmDetection 프레임웍이 알아서 호출함.
src Dataset에서 Middle format을 활용하여 trn/val/tst용으로 각각 Dataset을 만든다.
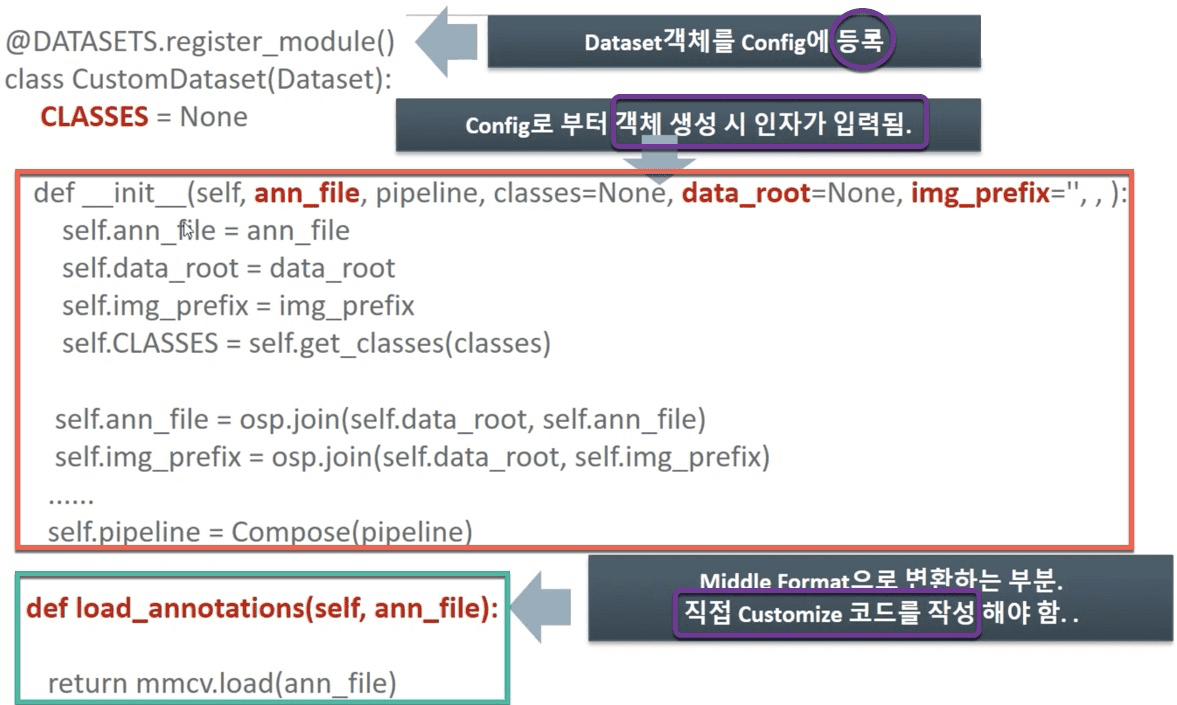
위 CustomDataset을 상속받아서…
filename, width, heights, ann을 key로 갖는 dictionary를 이미지 갯수대로 가지는 list를 <메모리상에> 생성
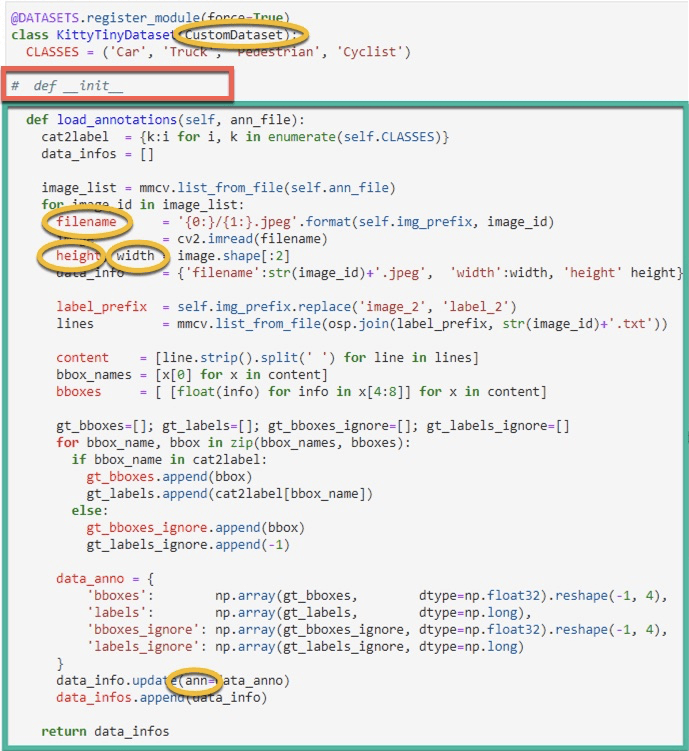
Config 변경 : Dataset 등록
Config 내용주석 : https://mmdetection.readthedocs.io/en/latest/tutorials/config.html#an-example-of-mask-r-cnn
mmdetection은 (code기반이 아닌) config기반이다.
config 와 dataset의 상호작용을 이해
https://github.com/open-mmlab/mmdetection/blob/master/mmdet/datasets/custom.py
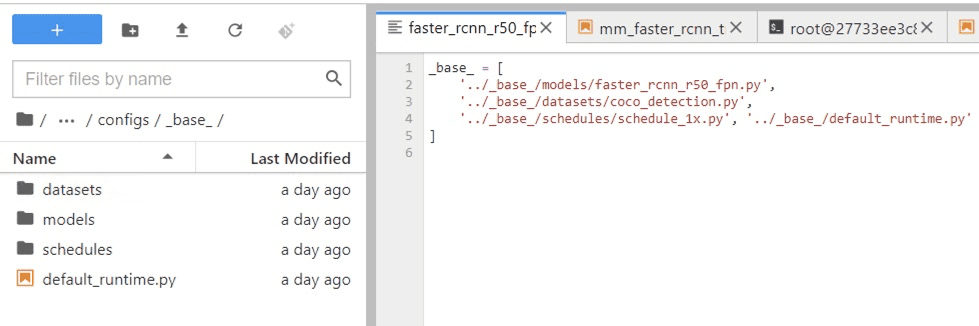
Dataset
Dataset (Image + Annotation) => Data Pipeline
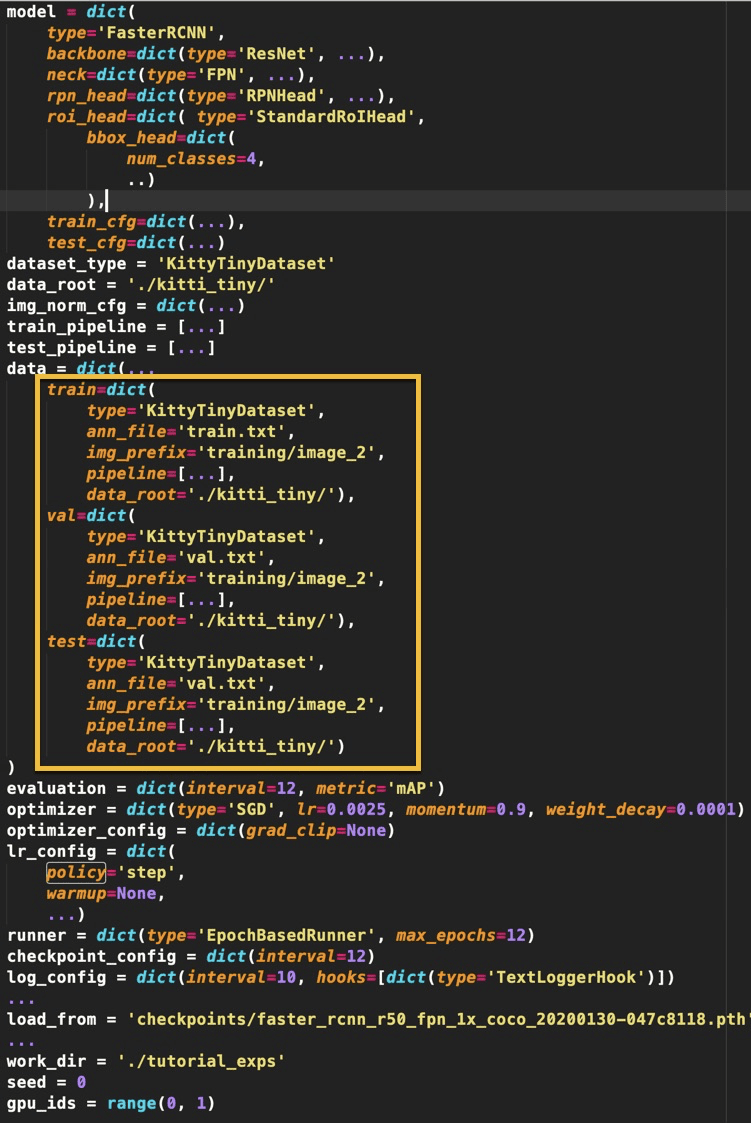
Custom Dataset 등록 및 메모리에 middle format dataset 만듦
- 아래 코드를 통해 CustomDataset 객체가 mmDetection 프레임웤에 등록되고, register_module()
- Config의 설정값(소스데이터 구조에 관한 data_root, img_prefix, ann_file)으로 객체가 생성되면, build_dataset()
- mmDetection 프레임웍이 알아서 호출함.
변환클래스를 통해 다양한 Dataset(Custom, Coco, Kitti, VOC, WIDERFace, XML데이터셋)을 지원
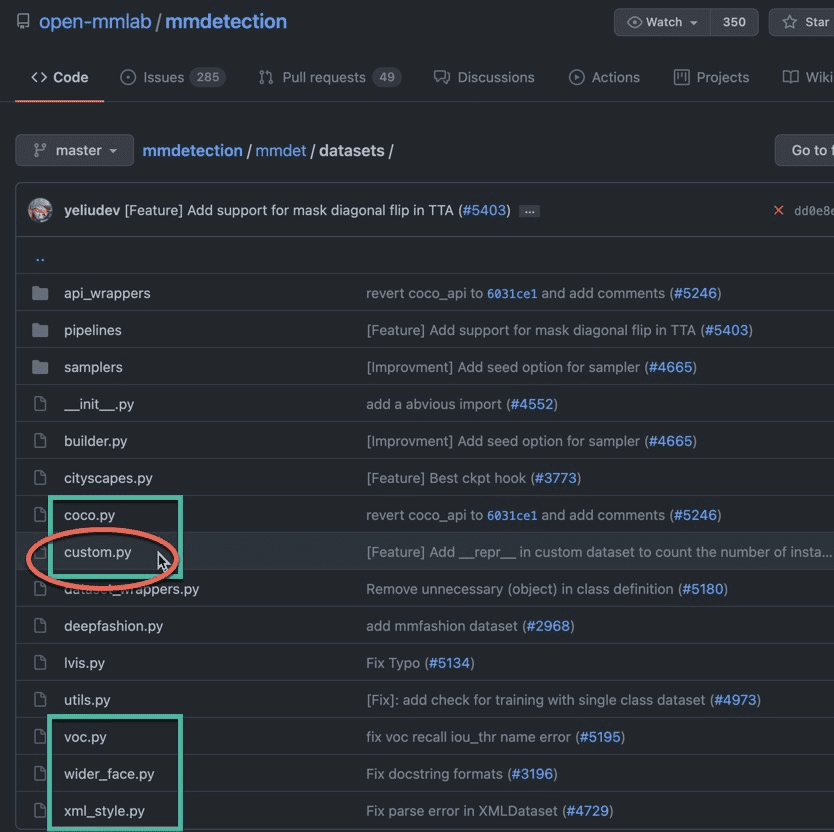
Data pipeline
https://mmdetection.readthedocs.io/en/latest/tutorials/data_pipeline.html
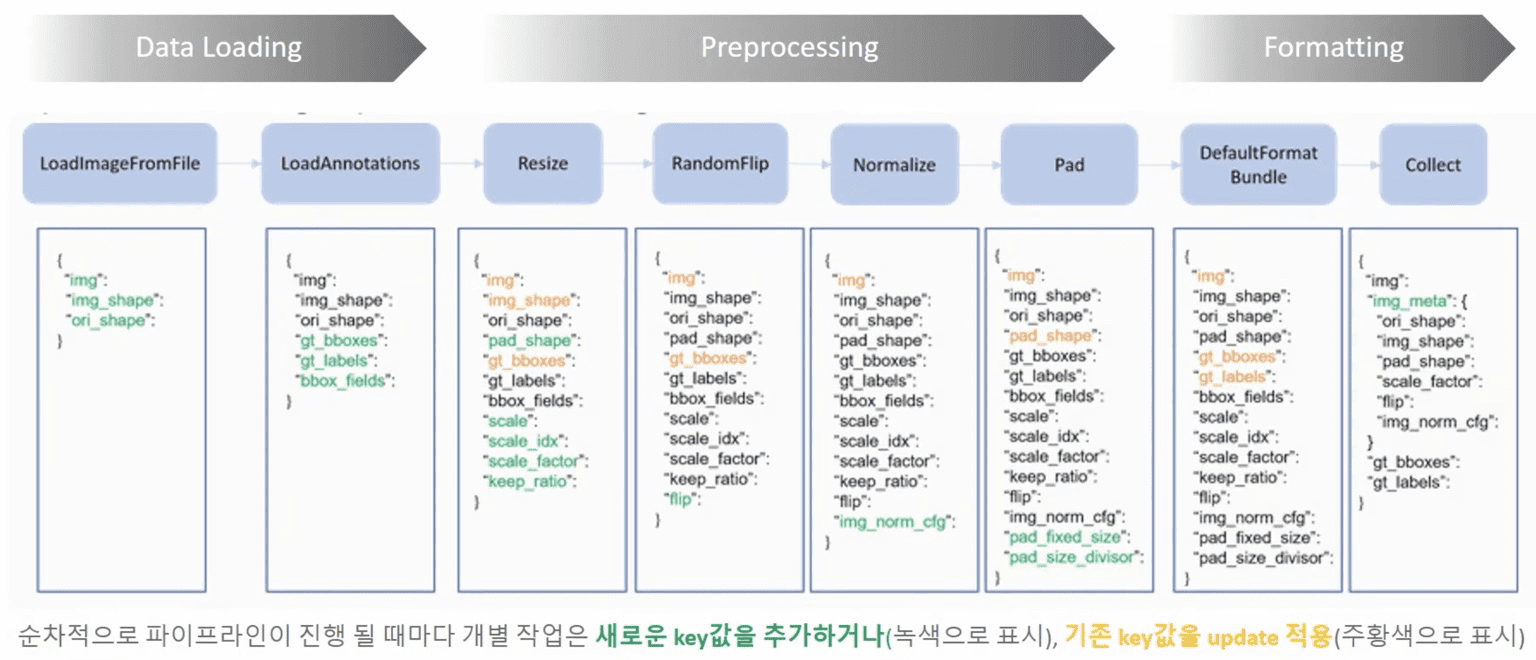
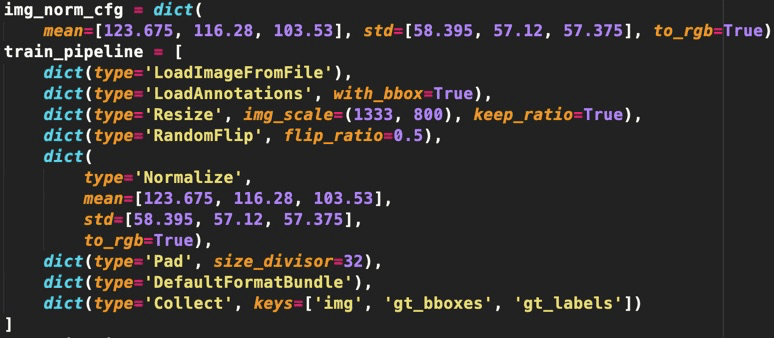
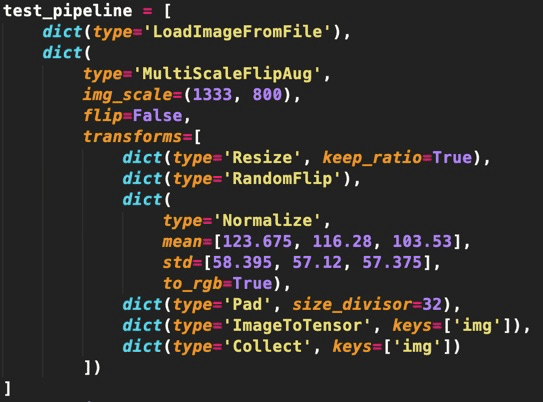
주어진 데이터셋의 구조를 파악 –> parsing –> mmdetection의 중립 annotation 포맷 변환
Model
roi head의 bboxhead 내에 Num_classes정도만 바꾼다.
Schedule
Tuning 포인트 ( 학습중 동적 learning rate)
runtime
Callback설정
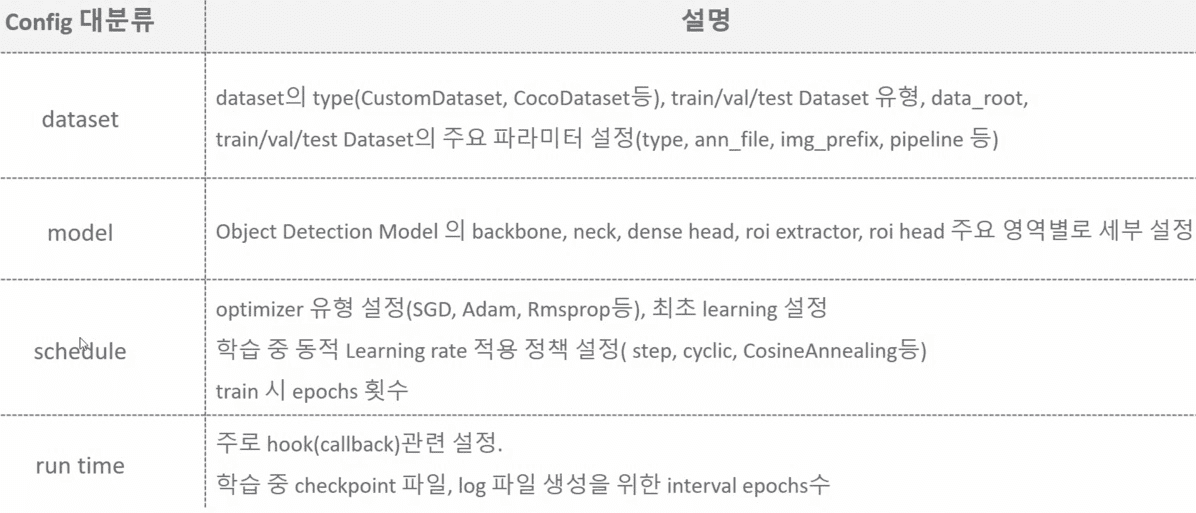
Train용 데이터를 생성하고 학습수행.
- [build_dataset()]로 train config 설정에 따른 Train용 Dataset 생성.
- build_detector()로 train과 test config반영하여 Model 생성.
- train_detector()로 Model 학습.
inference
학습된 model을 이용(Checkpoint 파일 로딩)하여 inference 수행
- 단일/여러 이미지 inference
test데이터로 Inference수행
- build_dateset()
- build_dataloader()
- init_detector()로 저장된 Model파일과 updated된 config를 사용하여 모델생성
- MMDataParallel()
- single_gpu_test() 결과가 시각화된 이미지로 반환됨.
- dataset.evalutate()
설치
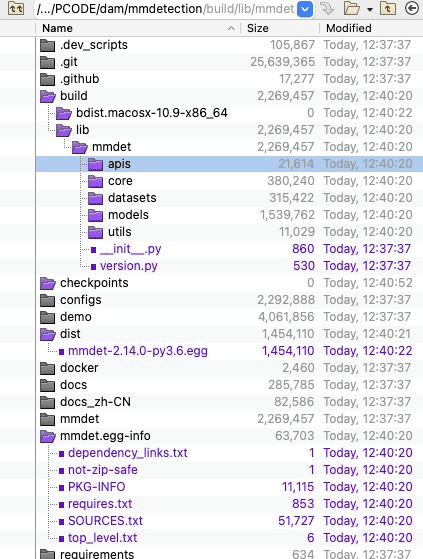
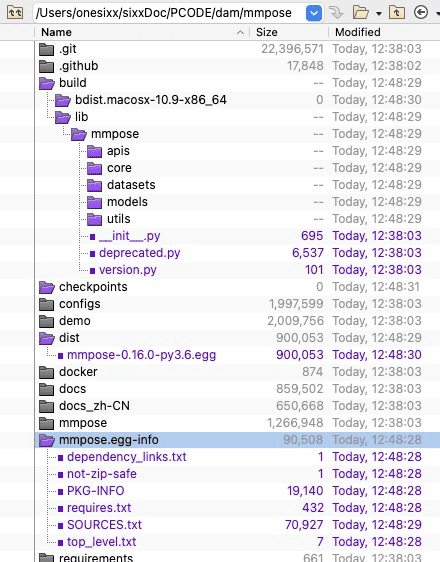
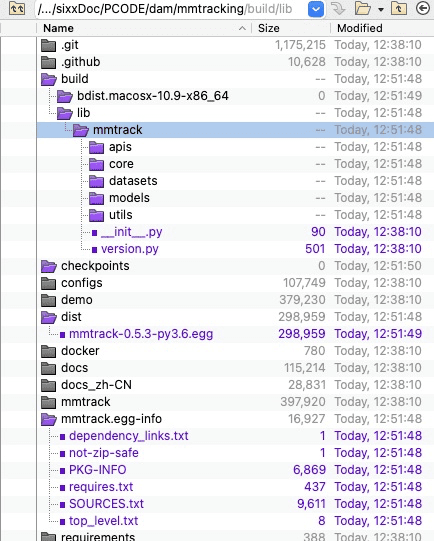